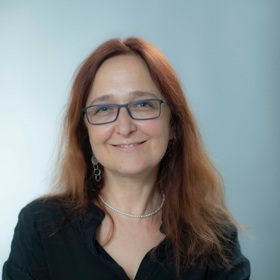
ד"ר יהודית אפרשטיין
-
apersteiny@afeka.ac.il
-
03-7688748
-
בניין הפיקוס, פיקוס קומת כניסה, פיק201
ד"ר יהודית אפרשטיין הינה מומחית בתחומי מערכות תבוניות ובינה מלאכותית. ב-15 שנים האחרונות הובילה ד"ר אפרשטיין עשרות רבות של מחקרים יישומיים במסגרת אקדמית, בשיתופי פעולה עם גופים שונים וכיועצת בתעשייה. ד"ר אפרשטיין מתמחה בבניית מערכות מורכבות שבדרך כלל משלבות מספר רב של רכיבים מבוססי AI שצריכים לעבוד ביחד תחת הרבה אילוצים של העולם האמיתי. המחקרים שמובילה ד"ר אפרשטיין עוסקים בפתרונות עבור יישומים במספר רחב של תחומים: תחזוקה חזויה, רפואה, ניתוח תמונות אוויריות, ניתוח נתוני חיישנים ואודיו. ד"ר אפרשטיין זכתה במספר מענקי מחקר מגופים שונים.
ד"ר אפרשטיין הינה בעלת תואר שני בתורת המשחקים מהטכניון, דוקטורט בכלכלה מתמטית ממכון ויצמן, עשתה פוסט דוקטורט בבר-אילן ופוסט-דוקטורט באוניברסיטת תל אביב. ד"ר אפרשטיין הינה בעלת תעודת הוראה ובוגרת תוכנית דו-שנתית בכלכלה ומנהל עסקים במכון ויצמן.
ב-2015 הקימה ד"ר אפרשטיין תואר שני במערכות תבוניות במכללת אפקה וכיהנה כראש התוכנית עד 2023.
למידה חישובית
סמינר בלמידה חישובית
למידה חישובית
ראיה ממוחשבת ורובוטיקה
למידה עמוקה
מערכות מרובות סוכנים
סמינר מערכות תבוניות ערים חכמות
2023, Afeka-Ariel Fund, Toward Breakthrough in the Field of Renewable Energy: AI-Based Decision Support System for Harnessing the Potential of Halide Perovskites, Principal Investigator, A joint project with Dr. Lena Yadgarov
2022, Mafat, Robust Deep Learning Model using Curriculum Learning, Principal Investigator
2021-2022, Mafat, Accuracy vs. Computation Cost in Distributed Deep Neural Networks Principal Investigator
2020, Mafat, Detecting structural faults in helicopters using artificial intelligence, Co-Researcher, A joint project with Makienko I., Gildish E. and Dr. Grebstein M.
2014-2017, Mafat, “Consumer brain computer interface devices for continuous authentication” Principal Investigator, A joint project with Prof. Anat Ratnovsky
2012-2013, Ben-Gurion University, Homeland Security Research Institute, “Web Text Mining for Monitoring Social Resilience”, Principal Investigator, A joint project with Prof. Yuval Cohen
2012-2013, Mekorot Water Technologies Entrepreneurship Center,“Outlier detection for water quality monitoring”, Principal Investigator with Dr. Dina Goren-Bar and Dr. Neta Rabin
2010-2011, Israel Chief Scientist ‘NeGeV” project, “Utility theory applied to social recommender systems”, Researcher, A Joint project with Dr. Dina Goren-Bar
Halevy, B. Y., Aperstein, Y., & Di Castro, D. (2023). Offline Skill Graph (OSG): A Framework for Learning and Planning using Offline Reinforcement Learning Skills. arXiv preprint arXiv:2306.13630.
Rokach, L., Aperstein, Y., & Akselrod-Ballin, A. End to End Active Learning Framework for Chest-Abdominal Ct Scans Segmentation. Available at SSRN 4502421.
Gildish, E., Grebshtein, M., Aperstein, Y., & Makienko, I. (2023). Vibration-Based Estimation of Gearbox Operating Conditions: Machine Learning Approach. In 2023 International Conference on Control, Automation and Diagnosis (ICCAD)(pp. 1-6). IEEE.
Lahiany, A., & Aperstein, Y. (2022). PTEENet: Post-Trained Early-Exit Neural Networks Augmentation for Inference Cost Optimization. IEEE Access, 10, 69680-69687.
Gildish, E., Grebshtein, M., Aperstein, Y., Kushnirski, A., & Makienko, I. (2022, June). Helicopter bolt loosening monitoring using vibrations and machine learning. In PHM Society European Conference (Vol. 7, No. 1, pp. 146-155).
Shavit, D., Davidovits, M., Kushnirsky, A., & Aperstein, Y. (2022). Temporal causality-based feature selection for fault prediction in rotorcraft flight controls. IFAC-PapersOnLine, 55(2), 235-239.
Aperstein, Y., Cohen, L., Bendavid, I., Cohen, J., Grozovsky, E., Rotem, T., & Singer, P. (2019). Improved ICU mortality prediction based on SOFA scores and gastrointestinal parameters. PloS one, 14(9), e0222599.
Bloch, E., Rotem, T., Cohen, J., Singer, P., & Aperstein, Y. (2019). Machine learning models for analysis of vital signs dynamics: a case for sepsis onset prediction. Journal of healthcare engineering, 2019.
Cohen Y., Aperstein Y. (2015) Service Oriented Acquisition Models for Serving Products with Short Expiration Period”, Series: Lecture Notes in Business Information Processing, Vol. 201, Springer. Proceedings, Exploring Services Science, 6th International Conference, IESS.
Aperstein, Y., Maymon, Y., Cohen, Y., & Singer, G. (2013). Nationality and risk attitude: Testing differences and similarities of investors' behavior in selected financial markets. Global Finance Journal, 24(2), 114-118.
Aperstein, Y., & Kannai, Y. (2006). Demand properties of concavifiable preferences. Journal of Mathematical Economics, 43(1), 36-55.
Aperstein, Y., & Holzman, R. (2003). The core and the bargaining set in glove-market games. International journal of game theory, 32, 189-204.
Apartsin, A., Aperstein, Y., & Gurvich, V. (1998). A circular graph—counterexample to the Duchet kernel conjecture. Discrete Mathematics, 178(1-3), 229-231.
יהודית אפרשטיין
שתף לינק באמצעות:
https://external.afeka.ac.il/faculty/יהודית-אפרשטיין/WhatsApp
Facebook
Twitter
Email
https://external.afeka.ac.il/faculty/יהודית-אפרשטיין/