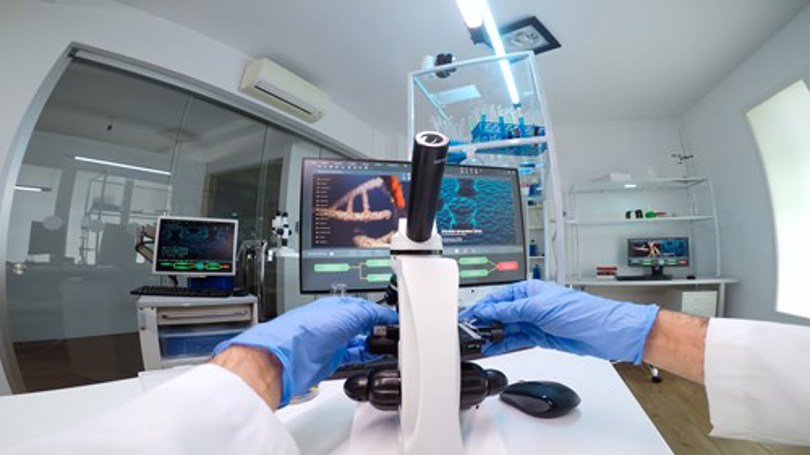
The article is based on a study that began as part of a prize-winning graduation research project by medical engineering alumnus Yuval Ashkenazi.
ABSTRACT: A Doppler ultrasound (DUS) is essential for detecting blood flow abnormalities in the umbilical cord (UC). Any morphological abnormalities of the UC may lead to morbidity and stillbirth. However, some morphological abnormalities such as torsion, strictures, and true knots may only be discovered at birth. This study proposes a novel approach for combining machine learning and blood flow velocity waveform analysis in order to improve the diagnosis of UC abnormalities.
Researchers designed and built a dynamic simulator that includes a flow sensor, a flow gauge, a water tank covered with an elastic membrane, and three umbilical cord models, each representing a different morphology. Using Doppler ultrasound, flow field images were captured from four cases of flow through the UC models. The ultrasound images were transformed by image processing into vector profiles of flow signals, and segmented into flow cycles for each of the cases. Flow cycle characteristics were extracted, and various machine learning algorithms were used to classify the different instances.
The study showed that the dynamic simulator designed by the researchers could produce an unlimited multi-case database, which in conjunction with machine learning could serve as a decision support system, increasing the ability to diagnose unseen UC pathologies.
Dr. Anat Ratnovsky and Dr. Sara Naftali published an article in Placenta
Share a link using:
https://external.afeka.ac.il/en/industry-relations/research-authority/dr-anat-ratnovsky-and-dr-sara-naftali-published-an-article-in-placenta/WhatsApp
Facebook
Twitter
Email
https://external.afeka.ac.il/en/industry-relations/research-authority/dr-anat-ratnovsky-and-dr-sara-naftali-published-an-article-in-placenta/